Urban Anomaly Detection
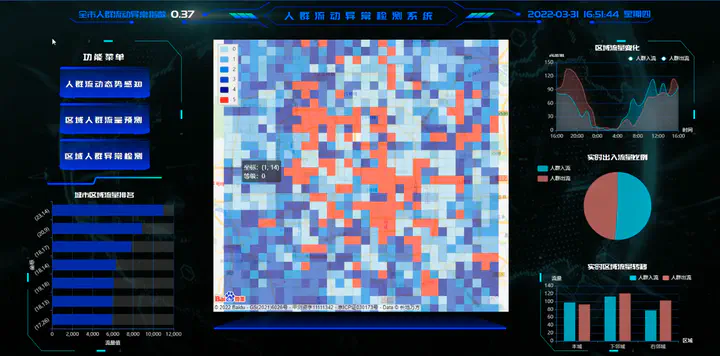
Urban anomalies, like traffic congestion and unexpected crowd gathering, usually occur in urban environments. It may pose tremendous risks to public safety and stability if not timely handled. For instance, on Apr. 30th, 2021, a tragic stampede took place in Mount Meron, Israel, resulting in 44 deaths and 150 injuries. If such urban anomalies could be timely sensed and detected in advance, the aforementioned accidents may be avoided. Accurate prediction of anomalies can help governments and communities properly deploy their limited resources to prevent anomalies from happening and ensure public safety.
-
State of Urban Crowd Flow Sensing: accurately describe the crowd flow in urban areas, including outflow and inflow, and show the amount of crowd transfer between regions.
-
Region Crowd Flow Predicting: employ deep spatio-temporal residual networks to citywide crowd flows prediction by modeling the temporal closeness, period, and trend properties of crowd flow.
-
Region Crowd Anomaly Detection: propse a dual channel Spatio-Temporal learning framework for Urban Anomaly Detection.